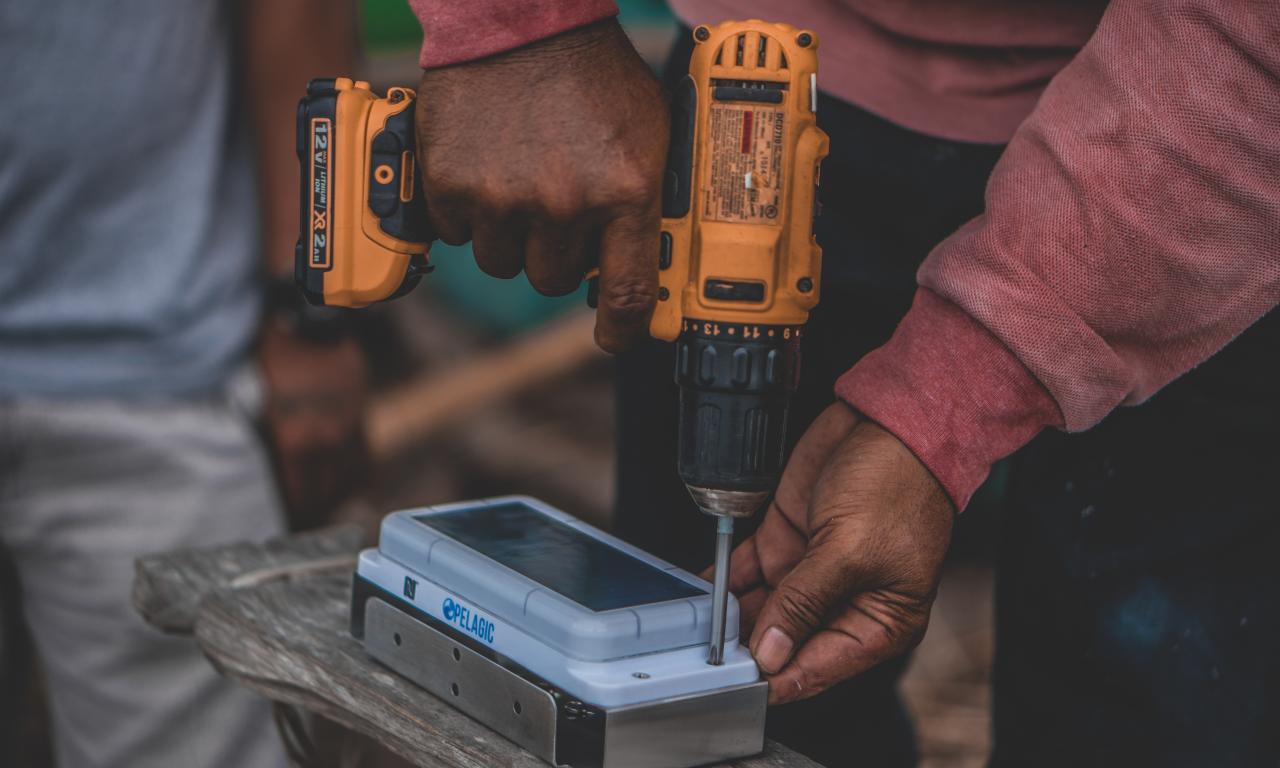
The digitalization of fisheries management holds much promise for productive, sustainable and inclusive aquatic food systems. However, the realization of these benefits depends on the availability of reliable and easily accessible data. This is particularly true in many parts of the developing world, which are plagued by significant data gaps.
A change is coming, though. A data revolution in SSF is already well underway in Timor-Leste, one of the newest, smallest and economically weakest countries in the world. Back in 2016, there was almost no reliable information about where coastal fishers went and what they caught. Less of a data gap and more of a gaping chasm, it meant the country’s policymakers had no evidence upon which to develop a fisheries strategy to improve food security, incomes and resilience to climate change.
The Peskas system changed that. Developed by scientists at WorldFish, in partnership with the country’s Ministry of Agriculture and Fisheries, it uses GPS trackers mounted on the boats of artisanal fishers to capture data about where they go, how often and for how long. Data collectors from the local community meet fishers as they come to shore, documenting their catch. The data is then uploaded to a publicly accessible online dashboard, providing a near real-time view of SSF in the country.
This kind of data can help policymakers decide where some fishing areas may need to be managed more sustainably, or where to deploy fish aggregating devices to improve catches. Peskas is now the official fisheries monitoring system in Timor-Leste and has been used to develop its latest National Fisheries Strategy.
Timor-Leste is now being hailed as an example of how Peskas can work in SSF in Kenya, Tanzania and Mozambique, as part of the WorldFish-led Asia–Africa BlueTech Superhighway (AABS) project, which started in 2023.
Bridging the Aquaculture Data Gap
But data gaps plague fish farming too. For example, until recently there was no baseline data to assess the performance of aquaculture systems, nor a standardized way of gathering it. This made it difficult to make recommendations for introducing or improving aquaculture technologies and practices—a huge challenge given its potential to provide nutrition in remote communities, particularly if agricultural crops fail.
To address this, under the CGIAR Initiative on Aquatic Foods, the WorldFish team undertook smartphone surveys in Nigeria, Egypt, Bangladesh and India involving thousands of fish farmers. These tracked productivity, profitability, input use and more. The results serve as benchmark data that can be used to show the benefits of different aquaculture approaches, from feeding regimens to the use of genetically improved carp and tilapia. Data from carp polyculture systems has already supported policymaking in Bangladesh and India.
Identifying data gaps in aquatic food systems requires comprehensive access to existing data. However, there is currently no organized integrated platform for scientists to do this. Nevertheless, a good place to start is FishBase, a free online database on marine fish distribution, traits, habitats and more. Due to the historical need for information to manage fisheries, FishBase is skewed toward commercially important marine fish species, so including a wider range of fish, especially those used in aquaculture, could strengthen the database.
Ensuring FAIR Data Standards
Although dealing with data gaps is essential, it is part of a bigger challenge facing scientists, and it is not just about the data. The real revolution hinges on the way data is presented and managed. Adhering to the FAIR principles—Findable, Accessible, Interoperable and Reusable—is essential for maximizing the usefulness of data.
Noise exists partly because of semantics and partly due to a lack of consistent standards in the way data is collected and managed. For example, a fish species can have many names in many languages, with researchers collecting information about it differently. This causes mismatches when scientists, or language-based artificial intelligence (AI) models like Chat GPT, try to compare catch data. One study might call it a “harvest” while another refers to “landings.” It means the data must be manually checked, which is a laborious task.
To tackle this, the Aquadata team is developing an ontology of SSF and aquaculture. This defines maps and categorizes the relations between different fish-related words and concepts to help clean up datasets. It is a simple housekeeping exercise for fish-related language, but one that will supercharge the data by making it FAIR and, therefore, ready for meaningful use by human and AI alike.
All in all, the data revolution in aquatic food systems is well on its way. With these foundations coming into place, fishers and fish farmers in some of the world’s poorest countries may soon benefit from the insights and opportunities the data revolution has always promised.
In some places, they already are.